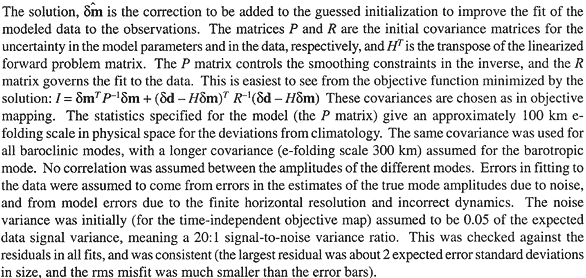
The matrix inverted in (3) is the time-dependent data-data covariance matrix, while P・HT is the model-data covariance matrix, so the result is equivalent to objective mapping with time-dependent covariance matrices.
At the end of each step, the nonlinearity of the forward problem is checked by comparing the data values predicted by the model using the new parameter estimates to the linearized approximation used for the inverse at that step. If the nonlinearities indicate that the assumed error levels are incorrect, the inverse can be repeated with larger data uncertainty to account for the nonlinearity. The iteration proceeds until either a fit is obtained within the expected data errors, or the nonlinearity cannot be reduced. If either the model or the forward problem is strongly nonlinear (with respect to the uncertainty in the initial guess), then it may be necessary to abandon the iterated linearization and use a global grid search or monte carlo method, in order to avoid local minima in the least-squares fit.
TIME-INDEPENDENT RESULTS
Before using the dynamical model, we do a series of estimations from 4-day average travel times. Figure 3 shows maps of these time-indepedent inverse results at 700 m (the depth of maximum perturbations), along with vorticity inferred from the triangles.
Figure 4 shows a comparison between the moored temperature data and the temperature field inferred from the time series of 4-day averaged inversions using acoustic data alone. The agreement shows the extent to which the large-scale tomography measurements have resolved the potentially small-scale thermal field. Use of time-dependent inversions to combine a longer duration of data should improve the resolution.
TIME-DEPENDENT RESULTS
Seven 12-day initialization experiments were run, starting at 1991 yearday 169, and continuing through yearday 241. These were chosen to be during the period with the most data, as some of the instruments lost transmitting power late in the experiment. The revised initializations fit the observations much